Perfectly "embedded": From tiny embedded MIPI cameras to ultra-compact all-in-one embedded vision systems.
Vision solutions that combine image acquisition and image processing in a compact design are becoming smaller and smaller, more and more powerful, and "embedded" in more and more applications, from classic machine vision systems and industrial quality control to factory automation and smart home appliances, to driver assistance systems and self-driving cars, autonomous robots and drones. In the process, the technology has developed rapidly over the past 30 years. Accordingly, the term "embedded vision" is used frequently today. In this blog post, we want to show what "embedded" means to us - and how numerous smart applications benefit from the technology.
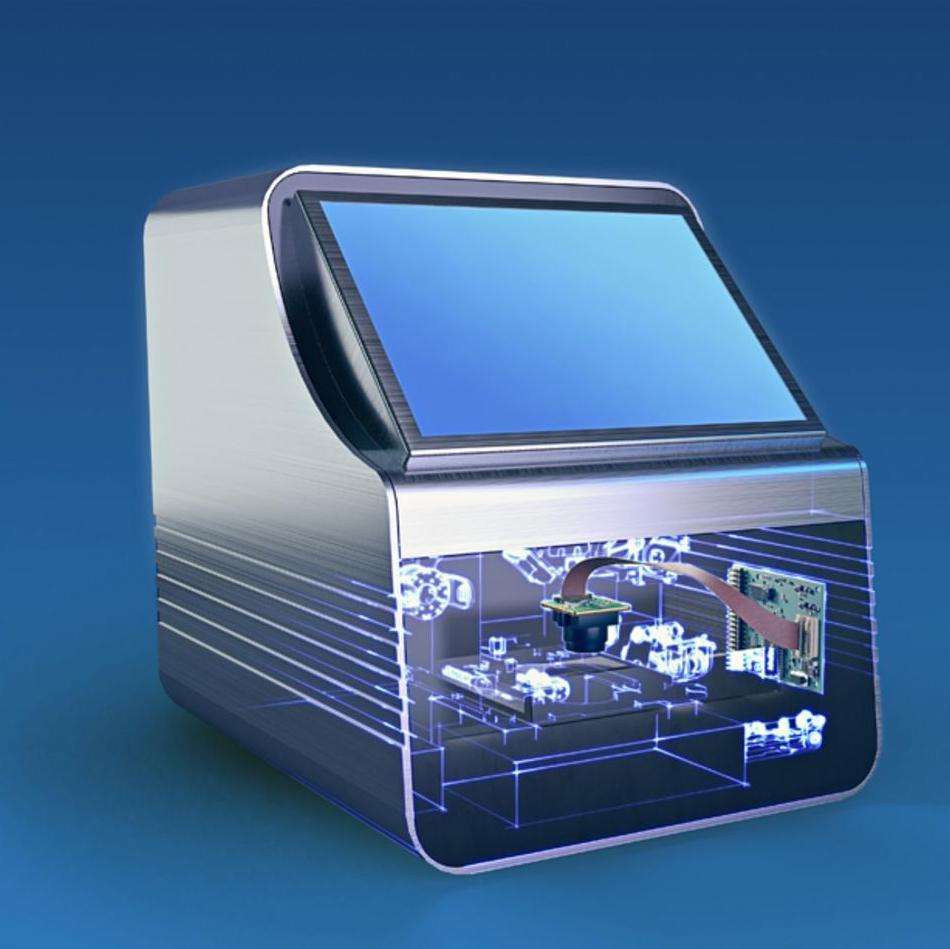
The Definition of an Embedded Vision System
Embedded systems usually refer to computer systems that combine a processor and memory as well as input/output peripheral devices, that have a dedicated function and that are implemented into larger mechanical or electronic systems (according to Wikipedia).
When it comes to embedded vision systems these solutions are usually based on an image sensor for acquisition of the data and a processing unit, for example a microcontroller. As cameras are being embedded as sensors in a growing number of applications, the label embedded vision is often used. A miniature board level camera module that can be deployed into various applications is often also referred to as an embedded camera or embedded vision component in the same way – and completely correctly – as are complex systems incorporating a camera module, a processing unit with mainboard and system on module and further electronics such as interface-boards, digital I/Os etc. Further components such as high-speed trigger, lighting and optics, other sensors and software can also be part of an embedded vision system.
Low power consumption is also an important aspect of embedded vision systems. In addition, rugged operating ranges and industrial-grade quality as well as long-term availability are also firmly linked to the term embedded electronics. The technology can be utilized and found in various applications from consumer end products to highly specialized industrial uses.
Basic components of an embedded vision system
Vision Components: Our take on embedded vision
" An important characteristic of embedded vision systems and their use in industrial and series products for VC are components that are perfectly tailored to the respective applications. They dispense with all unnecessary overhead in terms of components and functionality, thereby ensuring low unit costs. These solutions are as small as possible and ideally suited for the design of edge devices and mobile applications."
Jan Erik-Schmitt
Vice President of sales at Vision Components
Embedded Vision Systems – main criteria:
- Small size
- Low power consumption
- Low per-unit cost
- Rugged operation ranges
Examples of embedded vision systems
MIPI cameras
Flexible in use, compatible with common processor boards using the MIPI CSI2 interface, and available from VC with a wide variety of sensors: MIPI camera modules can be deployed in numerous embedded vision applications.
Camera modules and CPUs integrated
These intelligent board cameras combine image acquisition and image processing - either completely on an ultra-compact board or with one or more remote sensor heads for flexible integration into devices and machines.
Ready-to-use sensors based on embedded vision
Embedded vision systems from VC are also available in robust protective housings and, depending on the variant, with illumination or line laser modules for 3D triangulation applications. This makes them ready for immediate use or the ideal basis for individual OEM sensors.
Applications and tasks for embedded vision:
- 2D and 3D measurement
- Code reading: 1D (barcode) + 2D (data matrix)
- Contour detection: independent of rota-tional position and hidden objects
- Completeness control
- Positioning and Alignment
- Product inspection
- License plate recognition
- Parking management
- Access control
- Tool recognition
- Multi-camera applications
- Autonomous driving & ITS
- Drones
- Smart-City/ Smart-Traffic
- Robotics
- Laboratory Automation
Embedded vision in action: How applications benefit from intelligent image processing
High computing power of modern embedded processors, the efficient use of ARM cores, FPGAs and special processor units such as GPUs, IPSs, NPUs, etc., and all this combined with low power consumption and compact design: The possibilities with embedded vision systems are unlimited and already today they are used in all sectors and industries - even in applications that in the past were equipped with systems based on PCs. They are self-sufficient, dispense with all unnecessary components and, thanks to their small size, can be integrated almost anywhere. This means they offer decisive technical advantages and are ideal for use in edge devices, handhelds and mobile units. In combination with artificial intelligence, embedded vision systems are the basis for numerous innovative and smart applications that are currently emerging in areas such as smart farming & agriculture, intelligent traffic, smart appliances, smart factory & logistics, as well as in health, medical & life sciences.
Proven worldwide for over 25 years
Camera modules and vision systems from VC are successfully used in numerous industries and applications, in measurement technology, automation and quality assurance as well as in robots, intelligent household appliances and solutions for smart cities, in drones and special vehicles, in medical technology and laboratory equipment. Some projects and companies that rely on our OEM components for embedded vision are presented here:
Do you also want to benefit from embedded vision?
Then read on to find out how you can best integrate cameras and image processing into your project. And write us your questions!
We have compiled the three most efficient ways to integrate embedded vision in this continuing blog post. And look forward to helping you with your individual projects and challenges.